Model Using Brain Signals May Work as Early Warning System for Seizures
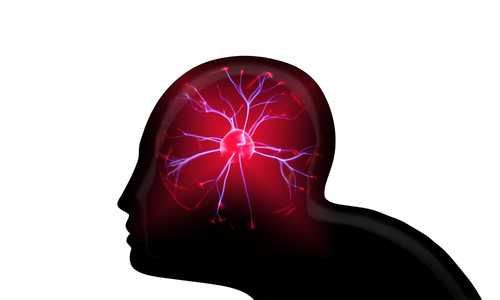
Researchers have developed a mathematical model to identify electrical signal patterns in the brain that predict epileptic seizures in advance, of benefit to people with seizure-related disorders such as Dravet syndrome, a study reports.Â
The study, “Early-warning for seizures could be a game-changer for epilepsy patients,” was published in the Journal of Neural Engineering.
Epileptic seizures result from a sudden surge of electrical activity in the brain. Seizures are not an actual disease but a symptom of various disorders that can affect the brain, including Dravet — characterized by seizure episodes lasting longer than five minutes and that are difficult to control with medications.
Scientists at the University of Southern California designed a mathematical model that uses electrical signals from the brain to give people who experience epileptic seizures a five- to 60-minute warning before seizure onset.
“For example, it could be as simple as just alerting the patient their seizure is coming the next hour, so they shouldn’t drive their car right now, or they should take their medicine, or they should go and sit down,” Dong Song, PhD, the study’s co-lead author, said in a press release.
“Or ideally in future, we can detect seizure signals and then send electrical stimulation through an implantable device to the brain to prevent the seizure from happening,” Song added.
The team worked with patients who had undergone intracranial electroencephalography (iEEG), in which electrodes were implanted, or placed directly on the surface, of the brain to record electrical activity in real time.
“Linear is the simple feature. If you understand the parts, you can understand the whole,” Song said. “Whereas the non-linear feature means that even if you understand the parts when you scale up, it has some emergent properties that cannot be explained.
“For some patients, linear features are more important, and for other patients, non-linear features are more important,” he added.
Furthermore, analyses of signals that occurred quickly, within milliseconds, were combined with those over a longer time scale.Â
“The brain is a multi-temporal scale device, so we need to understand what happens not just in the short term, but many more steps in the future,” Song said.
This way, the model is specific to an individual patient, as there are differences in preictal signals among people in advance of seizures.
Test results showed the model accurately classified seizure states — five to 60 minutes in advance — using iEEG data recorded in 10 people and in canines. The team showed that adding a longer time scale and non-linear features significantly improved the model’s performance.
An analysis found a high degree of variability in the types of electrical signals that contributed to seizure prediction across different patients.Â
“Epileptologists are still relatively few in number in many parts of our country and world. While they can identify many subtle features on EEG, the kinds of models that Song can create can identify additional features at a massive scale necessary to help the millions of patients affected by epilepsy in our region and worldwide,” said Charles Liu, MD, PhD, the study’s other co-lead author.
An unexpected potential benefit of this approach emerged from the COVID pandemic, which greatly affected epilepsy treatment. Patients whose epilepsy cannot be controlled by medication are often admitted to the hospital for EEG monitoring. With the pandemic, these elective admissions completely stopped, and epilepsy programs discontinued.
Applying the model using EEG recordings from the scalp or spinal electrodes can potentially be done at home and analyzed computationally.
“This is hopefully, going to change the way we deal with epilepsy going forward, and it’s driven by the needs that have been in place for a long time but have been highlighted and accelerated by COVID,” Liu said.
“We need to create a new workflow by which, instead of bringing patients to the ICU, we take the recordings from their home and use the computation models to do everything they would have done in the hospital,” Liu added. “Not only can you manage patients using physical distancing, you can also scale in a way that only technology allows. Computation can analyze thousands of pages of data at once, whereas a single neurologist cannot.”
Overall, the study “suggests that seizure generation may involve distinct linear/non-linear dynamical processes caused by different underlying neurobiological mechanisms,” the team wrote. “It is necessary to build patient-specific classification models with a wide range of dynamical features.”
Christianne Heck, MD, a study author, noted two important issues to this technology’s relevance.
“One is that a majority of patients who suffer from epilepsy live with fear and anxiety about their next seizure, which may strike like lightning in the most inopportune moment, perhaps while driving or just walking in public. An ample warning provides a critical ‘get safe’ opportunity,” Heck said.
“The second relevant issue clinically is that we have brain implants, smart devices, that this engineered technology can enhance, giving greater hope for efficacy of our existing therapies.”