Artificial Intelligence May Speed up Epilepsy Diagnoses, Study Suggests
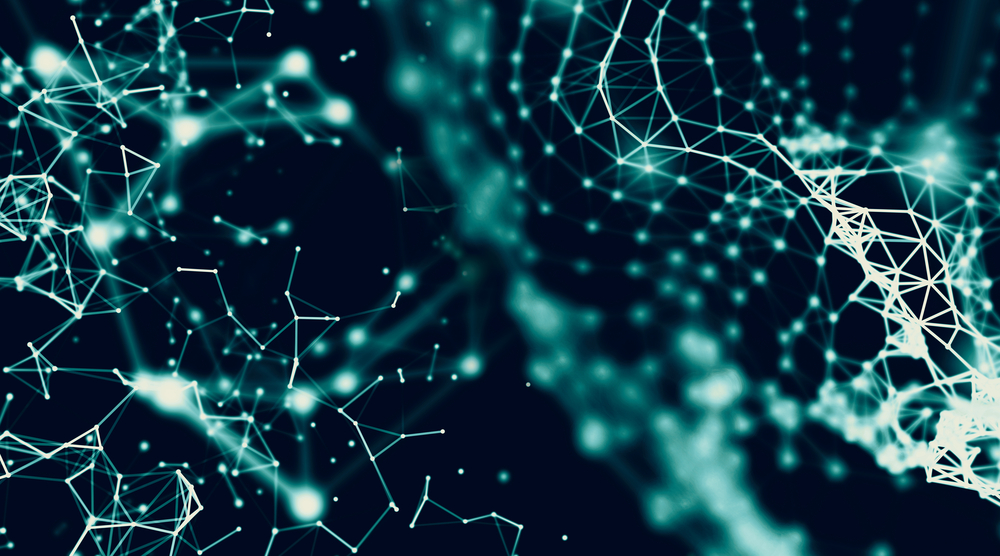
A newly developed artificial intelligence (AI) system could help expedite the diagnosis of epileptic conditions such as Dravet syndrome.
The AI system was described in a study, titled “A propositional AI system for supporting epilepsy diagnosis based on the 2017 epilepsy classification: Illustrated by Dravet syndrome,” in the journal Epilepsy & Behavior.
Epilepsy is a broad disease category for many different conditions that involve seizures. Properly diagnosing epileptic conditions can be a challenge, especially given their different causes and symptoms.
For example, mutations in the SCN1A gene are the most common cause of Dravet syndrome, but not all people with Dravet syndrome have such mutations, and SCN1AÂ mutations can also be associated with other conditions, such as febrile seizures plus.
The International League Against Epilepsy (ILAE) established guidelines for classifying epilepsy in 2017. The framework emphasizes determining the underlying cause of seizures, and taking into account comorbidities — the presence of additional medical conditions — such as psychiatric disorders.
However, using the guidelines to make a diagnosis requires synthesizing a large volume of information including genetic tests, family history, specific seizure types, and age of onset. This makes diagnosis difficult, especially with the ever-expanding knowledge about these conditions.
In the new study, researchers created an AI system to aid in the diagnosis process. Conceptually, the program is fairly straightforward: first, data about an individual is fed into the program. Then, the program searches through detailed databases to find the condition that best matches the individual’s data.
“For example,” the researchers wrote, “if the only type of seizure is epileptic spasm and the first seizure occurs when the patient is younger than 1.5 years old, we will consider the possibility of the patient having West syndrome. For another example, an early childhood onset seizure with myoclonic-atonic seizure should be considered Doose syndrome.”
In these examples, relatively little information is needed to make a diagnosis. The same logic applies for other conditions, but with more information taken into account.
The researchers illustrated the utility of their system using the case of an 18-year-old male patient who was clinically suspected of having Dravet syndrome at age 5 and diagnosed at the age of 10, after a history of seizures that started when he was 7 months old. The researchers showed that when the program used only age of onset, the patient was given many possible diagnoses. As more of the patient’s clinical features (seizure types, etc.) were inputted into the program, the number of possible diagnoses decreased, until the only remaining condition was Dravet syndrome.
“The purpose of the illustrated case is to show the whole picture on disease progress of the patient with typical Dravet syndrome,” the researchers wrote. “[F]or each new case of Dravet syndrome, we believe that with the recommendation of the AI system, a doctor can make an earlier diagnosis by reliably narrowing down the differential diagnosis, when the patient developed typical seizure types, [electroencephalogram] patterns, speech delay, and psychomotor retardation.”
This AI tool may help physicians diagnose epileptic conditions with greater ease and speed. However, the system is not without limitations: mainly, the AI is reliant on having accurate and up-to-date data in its database to make correct diagnoses, so the system will have to be regularly maintained and updated if it is to have future utility. Additionally, the researchers noted that the system requires further testing, particularly on “borderline” cases that are diagnostically challenging.
“We constructed a propositional AI system based on the new ILAE epilepsy diagnostic framework and the most updated epilepsy-related etiology data,” the researchers wrote. “Through the current system and inference engine application, diagnoses of epilepsy syndromes were inferred from relevant information and parameters of patients with epilepsy.”