Multimodal Analysis is Best to Categorize SCN1A Genetic Variants, Study Says
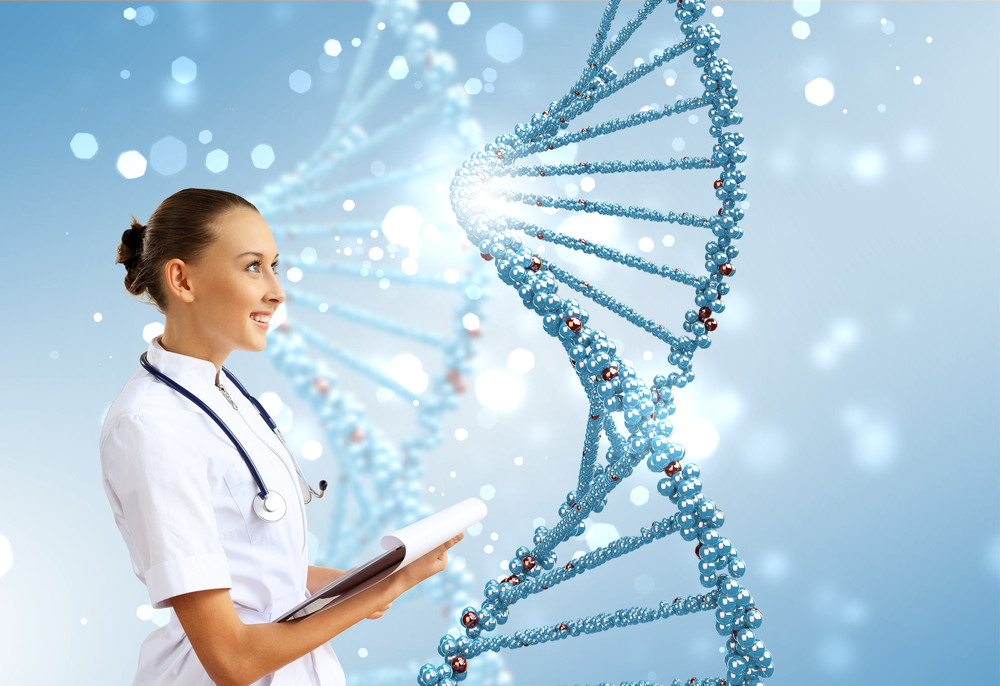
Multimodal analysis that incorporates data obtained from different methodologies seems to be the best approach to build a solid classification system for SCN1A genetic variants associated with Dravet syndrome, a study says.
The study, “Multimodal analysis of SCN1A missense variants improves interpretation of clinically relevant variants in Dravet syndrome,” was published in Frontiers in Neurology.
Dravet syndrome (DS) is a severe type of epilepsy that usually manifests during the first year of life and is characterized by seizures, cognitive deficits, and increased mortality. In most cases (70-80%), the disorder is associated with known genetic mutations in the SCN1A gene, which encodes for a sub-unit of a sodium channel called NaV1.1, responsible for the transmission of electrical signals in the brain.
“Recent scientific and technical breakthroughs have allowed for the increasing use of genetic testing in clinical practice, providing more precise diagnoses and having significant prognostic and treatment implications. However, interpreting the clinical significance of genetic variants found in molecular tests can still be challenging, particularly for variants of uncertain significance (VUS; genetic variants whose biologic impact is unknown),” researchers stated.
To incorporate the contribution of VUS on disease development, a group of Brazilian scientists set out to create a new classification system for all missense (single nucleotide mutation that alters protein composition)Â SCN1A genetic variants associated with Dravet syndrome. Nucleotides are the building blocks of DNA or RNA.
Researchers combined and modified classification criteria that had been proposed by the American College of Medical Genetics and Genomics (ACMG) and the Association for Molecular Pathology (AMP) in an attempt to minimize the frequency of inconclusive test results. These criteria included information on protein alterations, patterns of inheritance, allele (variant forms of the same gene) frequencies in specific populations and estimated negative effects on protein function.
The new score classification system was based on pathogenicity (the ability to induce disease) associated with each genetic variant. Information on SCN1A genetic variants was gathered from the literature and from a group of 21 individuals with Dravet syndrome who were examined and classified according to the new classification system.
SCN1A mutation screenings revealed that 81% of the patients participating in the study carried a potentially harmful genetic variant. All 16 potentially pathogenic mutations were found in heterozygosity (each patient only carried one copy of the mutated SCN1A gene) and were stored in the Brazilian Initiative on Precision Medicine database.
In combination with all SCN1A genetic variants reported in the literature, researchers found 1,101 potentially harmful mutations in patients with Dravet syndrome. Meta-analysis results showed that 46% of the variants were missense mutations.
After applying the new classification system, 56.5% of the potentially harmful mutations changed classification from variants whose biologic impact is unknown to “pathogenic” (17.8%) or “likely pathogenic” (38.7%).
“[O]ur results indicate that to better interpret the pathogenic impact of missense changes in SCN1A in the context of the molecular diagnosis of patients with [Dravet syndrome], the use of multimodal analysis seems to be the best approach, since no single method can unequivocally classify all variants found,” the researchers said.
“The combination of these strategies seems to improve the accuracy of prediction and the likelihood of offering a clinically relevant report to clinicians as indicated by our results showing that by applying the proposed modified workflow, most DS-related SCN1A variants had their classification improved,” they concluded.